
BLOG
Quloud-Mag Explanation (2) Technologies provided by Quloud-Mag
In this second part, we will explain the difference between conventional magnetic material simulation and the "multi-scale simulation" provided by Quloud-Mag.
INTRODUCTION
MULTISCALE
Multi-scale simulation for magnetic material development provided by Quloud-Mag
Quloud-Mag provides the world's only multi-scale simulation for the development of new magnetic materials. Quloud-Mag has succeeded in connecting first-principles material calculations and empirical material simulations, extracting parameters for multi-scale simulations from first-principles material calculations, and using the extracted parameters to perform empirical material simulations, making it the world's only simulation software that can simulate the behavior of magnetic materials at finite temperatures and the magnetic properties of nanoparticles without experimental values. In addition, the software also provides a technology called data assimilation technology, which will be explained later.
The figure shows the simulation flow. First, enter the crystal structure of the material you want to simulate. You cannot start the simulation unless you know the crystal structure information. First-principles material simulations run on the input information of the crystal structure. In first-principles material simulations, density functional (DFT) calculations are performed, and no information about the material other than the crystal structure is required. In density functional calculations, physical quantities such as magnetic moment and magnetic anisotropy at absolute zero can be calculated. Furthermore, three parameters necessary for the subsequent empirical material simulation, the exchange interaction Jij, the Jaron-Shinski-Moriya interaction Dij, and the Gilbert damping parameter, are obtained. The subsequent empirical material simulation consists of two parts. One is a Monte Carlo simulation, and the other is a micromagnetic simulation. First, the Monte Carlo simulation is explained. Using the quantities obtained in the first-principles material simulation, namely the magnetic moment, magnetic anisotropy, the exchange interaction Jij, and the Jaron-Shinski-Moriya interaction Dij, something called the Heisenberg Hamiltonian is constructed, and the empirical equation of motion is derived. According to the derived equation of motion, a simulation called a Monte Carlo simulation is performed, which incorporates the effect of finite temperature that was not taken into account in the first-principles material simulation. The temperature dependence of the magnetic moment, magnetization curve, magnetic susceptibility, magnetic anisotropy, specific heat, entropy change, adiabatic temperature change, and electrical resistance is simulated by the Monte Carlo simulation, which allows the Curie temperature and Neel temperature (the temperature specific to the material at which magnetic properties disappear) to be obtained. Only at this stage can the behavior of magnetic materials at room temperature be predicted. However, the size that can usually be handled by Monte Carlo simulations is at most about 10 nanometers. On the other hand, another important factor that must be taken into account when calculating the magnetic properties of magnetic materials is the magnetic domain wall. In magnetic materials, a certain region or domain where the magnetic moment is aligned is called a magnetic domain, and a magnetic structure called a magnetic domain wall is formed at the boundary of the magnetic domain, and it has a structure like a "wall" that separates adjacent magnetic domains. When a magnetic field is applied from the outside, this magnetic domain wall moves, and the size of each magnetic domain changes, indicating the response to the external magnetic field. Therefore, the ease of movement of this magnetic domain wall is one indicator that affects the magnetic properties. The easier the magnetic domain wall moves, the faster the response to the external magnetic field. In simulations, the ease of movement of this magnetic domain wall must be taken into account. By the way, the typical scale of a magnetic domain is about 100 nanometers to 1 micrometer, and if you try to simulate magnetic properties taking into account the movement of the magnetic domain wall, you need to perform a simulation of a size of several micrometers. As I mentioned earlier, current computers can only handle up to about 10 nanometers in Monte Carlo simulation, so we need to run a simulation on a slightly larger scale. Therefore, we need to connect to micromagnetic simulation, which is an empirical simulation on an even larger scale. In fact, the exchange stiffness, which is a parameter output from the Monte Carlo simulation, and the Gilbert damping, which is a parameter output from the first-principles material simulation, are input, and the micromagnetic simulation is run. In micromagnetic simulation, it is possible to simulate magnetic properties including the movement of domain walls, and physical quantities such as hysteresis curves and complex permeability are simulated. In particular, in recent years, this complex permeability has become an important physical quantity for energy-saving device design and has attracted a lot of attention. In addition, because Quloud-Mag is a multi-scale simulation, it is a material simulation software that can simulate physical quantities such as complex permeability from the first principles, making it unique in the world. To what extent this reproduces the experimental values, please see the actual calculation results in Part 3.
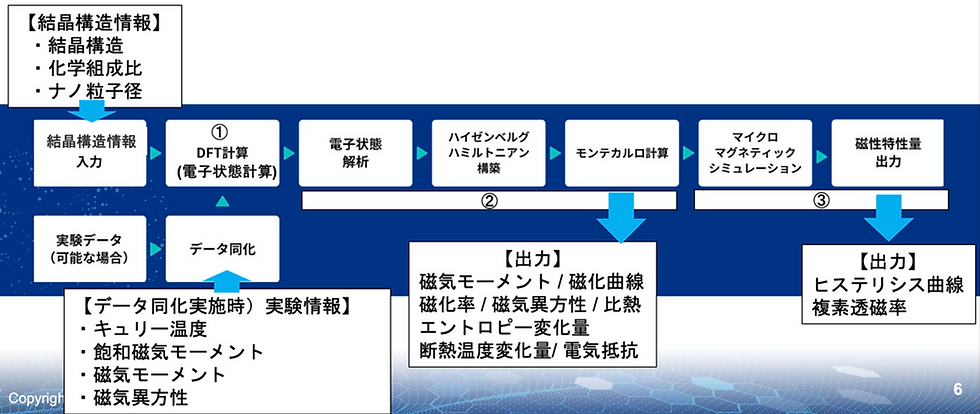
ASSIMILATION
Another feature provided by Cloud-Mag: Data assimilation technology
We have explained that Quloud-Mag is the only software in the world that connects first-principles simulation with empirical simulation. Here, we will explain another important function of Quloud-Mag, data assimilation technology. Quloud-Mag uses density functional theory for first-principles material simulation. This density functional theory is known to be highly accurate, but because it uses approximations, it is not possible to achieve perfect agreement with experiments. For example, the Curie temperature can deviate by up to 20%. In reality, it is amazing that it only deviates by 20%. In many cases, we are interested in the "relative" behavior of the Curie temperature, such as how it changes when impurities are added, so even if there is such a deviation, it is usually used to predict new materials by focusing on the relative behavior. However, sometimes there is a need to know a certain physical quantity of a certain material that has already been synthesized experimentally, "as quantitatively and accurately as possible." The function that can be used in such cases is data assimilation technology. When relatively easily obtained experimental values, such as the Curie temperature or the magnetic moment at a certain temperature, are input, the input parameters of the empirical material simulation are fed back so that the simulation results are consistent with the input experimental results. This allows the experimental results to be reproduced, improving the accuracy of the entire simulation. Importantly, once some physical quantities are updated to reproduce the experiment, the effect extends to other physical quantities, and the accuracy of those quantities also improves significantly. Data assimilation technology was originally introduced in weather forecasting, and was developed as a technique to improve the accuracy of simulations.
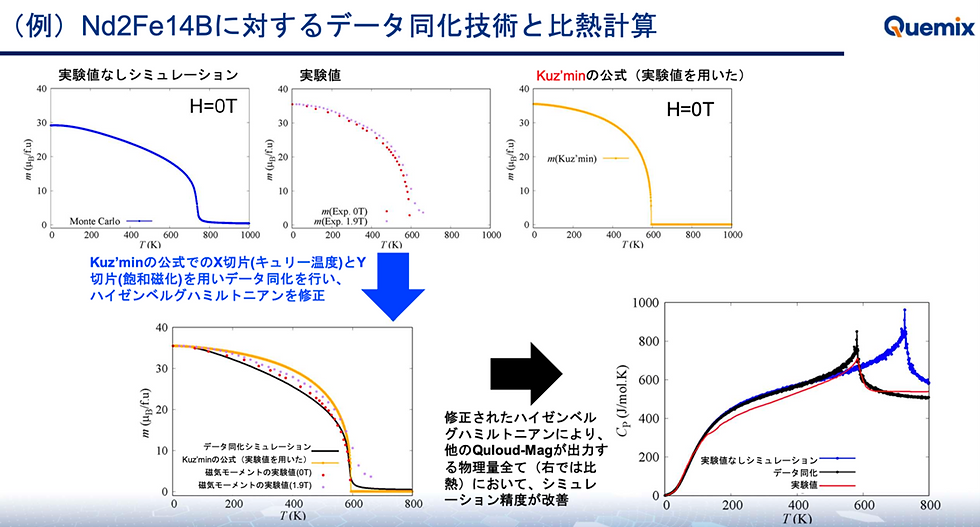